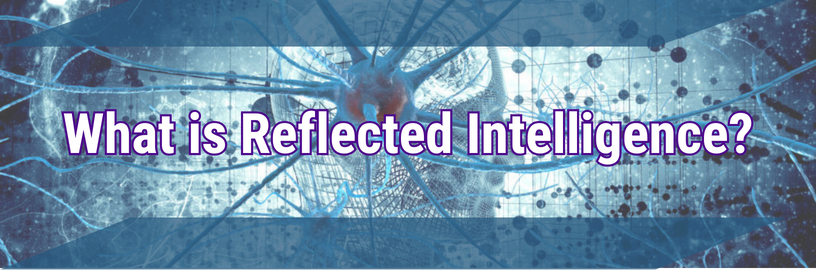
What Is Reflective Intelligence?
By Chance Whittley, Principal AI Consultant, PTP
Gen AI and the Subjective Customer Experience
In the rapidly evolving digital landscape, the power of Generative Artificial Intelligence (AI) is undeniable, yet its potential is often undermined by a critical oversight: the neglect of the customers’ subjective experience. Enterprises deploying one-size-fits-all solutions fail to engage customers effectively, overlooking the nuances of human communication and their own brand identity. This not only diminishes the customer experience but also hampers the success of an Enterprise’s Generative AI initiatives. Without incorporating Reflected Intelligence (RI), these systems overlook enterprise-specific knowledge, processes, and branding, which are crucial for meaningful AI interactions.
Enhancing Gen AI with Reflected Intelligence
Reflected Intelligence (RI) is a solution that bridges this gap, ensuring that Enterprise Generative AI fulfills its promise of transforming business operations. RI goes beyond technical proficiency by mirroring an organization’s human intelligence and capturing employee knowledge, communication styles, and company culture. By understanding the customer and enterprise context, RI customizes responses, fosters trust, and drives adoption. This article explores the core principles, benefits, and implementation strategies of RI, paving the way for a new era of customer service-centric AI experiences.
The Problem with AI
Experience experts have emphasized the technological potential of personalization and efficiency through Generative AI, overlooking the critical aspect of how customers perceive their interactions with AI systems subjectively. This standardized approach, where AI interactions remain inherently neutral and objective despite being augmented with human-like conversational elements and response indicators, results in experiences that can feel unengaging and detached from the brand identity customers have come to expect. Such generic interactions, rooted in limited data sets and an absence of contextual understanding, fail to capture the subtleties of human communication and knowledge, thereby impeding user adoption and satisfaction.
There are two basic types of AI models:
1. Discriminative AI models are designed to classify and categorize data based on their training data sets. These models are often used for predicting the next word in a sentence, classifying information, and following predetermined steps. The output of discriminative AI models is selected from the existing options provided in workflows or datasets. These models are commonly used for tasks such as routing calls, sentiment analysis, chatbot interactions, IVR NLU, and spam filtering.
2. Generative AI models can combine reasoning with content creation (text, voice, or images) to convey accurate understanding and results to the end user. These modules are commonly used for various applications, such as IVR Natural Language Understanding (NLU), Generative Agents or Assistants for Voice and Chat, and Generative Analysts for Voice, Text, and Data.
The Solution
RI addresses this gap and is a transformative solution enabling AI to mirror an organization’s employee knowledge, communication style, and culture. RI aims to elevate the customers experience, fostering a more personalized and engaging interaction that resonates with the enterprise’s identity and values. This integration challenges the prevailing norms of AI interactions and signifies a pivotal shift toward creating enterprise-acceptable AI solutions.
RI Core Principles
At its core, RI allows AI to capture, understand, reflect, and mirror an enterprise’s peer-to-peer (employee) knowledge, existing business processes, common communication styles, and the enterprise’s culture. The result is to improve the customer’s experience when interacting with the AI, leading to increased adoption.
Employee Knowledge: RI captures the collective knowledge and expertise shared between employees during interactions. These are often situations and/or information not collected or captured properly within Knowledge Management databases. This type of intel may also discover employee practices still in use that are different from the preferred method of resolution.
- Business Processes: RI also expands the Generative AI’s Reasoning and Acting (ReAct) approach to better understand all the business processes and workflows in place, allowing the Generative AI to predict the following best action(s) within the desired process(es). This reflects how tasks are typically completed within the organization.
- Communication Styles: RI can also understand how employees communicate with each other and with customers, which is essential to maintaining the brand image and keeping people engaged in the interaction.
- Company Culture: Absorbing the overall corporate values, attitudes, and behaviors that define the company’s work environment is another benefit of AI. It enables an understanding of the organization’s decision-making process and further fine tunes a Generative AI’s ReAct approach.
RI Benefits
The concept of RI underscores the importance of automation being technically proficient but also being deeply integrated with and reflective of the organization’s specific context and nuances. It enables a more natural and helpful AI experience that will boost trust and adoption, leading to improved efficiency and return on investment. Some of the key benefits include:
Increased Knowledge: Bridge information gaps between departments by capturing and sharing tacit knowledge exchanged between employees.
- Context-Aware Responses: Consider the user’s role, department, and current task when providing information or completing requests, leading to more relevant and helpful responses.
- Increased Adoption: The adoption of RI models leads to AI interactions that are more relevant and engaging for both employees and customers.
- More Natural Interactions: By understanding communication styles and company culture, RI can tailor its responses to feel familiar and natural to customers, leading to smoother and more engaging interactions.
- Enhanced Brand Identity: RI ensures that AI interactions resonate with the brand identity and values, creating a more cohesive and satisfying customer experience.
- Increased Trust: customers are likelier to trust an AI that reflects their company’s values, understands their work and communication environment, and provides accurate information.
RI Challenges
It is important to acknowledge that any innovative technology comes with unique challenges that need to be considered beyond the typical concerns of privacy, confidentiality, risk, and compliance. However, a proactive and thoughtful approach can effectively address and manage these challenges.
- Passive knowledge: Captured from real-world interactions can sometimes lead to inaccurate or biased information. This, in turn, may require data cleaning and filtering techniques to be applied, which are typically carried out by technical specialists who are knowledgeable about the relevant tools and techniques. By using these techniques, it is possible to mitigate the impact of any unwanted biases or inaccuracies that may have been introduced during the knowledge capture process.
- Scalability and Adaptability: Scaling RI to handle large organizations with diverse departments and workflows can be complex. Additionally, adapting RI to evolving company cultures and communication styles requires ongoing maintenance and adjustments.
- Technical Implementation: Extracting meaningful insights from unstructured data (like conversations) requires technical specialists who can navigate the complexities of Generative AI models, reasoning systems, and unstructured data processing.
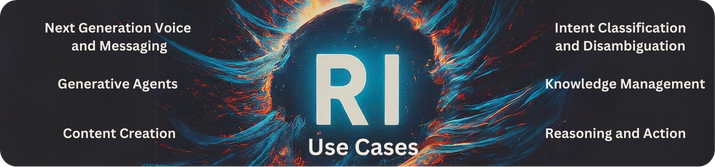
Implementation and Controls
To successfully implement an RI model, it is crucial to establish a strong partnership between business leaders and technical experts. They need to work together with confidence to define an overall strategy and roadmap that encompasses the right combination of innovative Generative AI tools and techniques and a robust architecture for data acquisition, processing, knowledge representation, reasoning, and multiple levels of safeguards and controls.
- Incorporate an “adversarial” Generative AI in real-time to fact-check the generative outputs before they are presented during an interaction. This adversarial Generative AI would act as a discerning reviewer or “Critic Agent,” identifying any potential biases, inaccuracies, or hallucinations produced, thus enhancing the overall accuracy and reliability of the system.
- To prevent biases and errors with RI, a human-in-the-loop (HITL) approach can be used. This involves presenting the captured data to human experts for validation before the Generative AI uses it. Human reviewers can check the data’s accuracy, completeness, and potential biases and make necessary corrections to increase the level of confidence.
Leverage Explainable Artificial Intelligence Techniques
- Feature Attribution to explain how individual pieces of data contribute to the AI’s final output.
- Model Visualization, which can help people understand the inner workings of the AI model, would outline the high-level steps for the critical decision elements.
- Counterfactual Reasoning allows people in the HITL feedback process to explore how the output would change if certain input features were modified.
Summing It Up
An RI model integrates seamlessly with business processes, reflecting an enterprise’s workflow, decision-making, and operational strategies. This allows it to begin absorbing and adapting to the enterprise’s culture and considering the prevalent value norms and behaviors. It also ensures that the interactions and outputs are relevant and appropriate.
- Generative AI’s focus on technological capabilities often misses the critical aspect of customer subjective perception, leading to interactions that fail to use enterprise knowledge, lack branding, and do not help customers.
- Standard AI approaches result in generic interactions that lack contextual understanding and do not capture the nuances of human communication.
- RI challenges the prevailing norms of AI interactions by integrating deeply with an organization’s unique identity and values. It aims to create AI solutions that are technically proficient but also meaningful and customer accepted.
- The adoption of RI models, designed to reflect and adapt to an enterprise’s culture and workflows, significantly improves customer experience. It also increases adoption by making AI interactions more relevant and engaging.
Current AI prioritizes technology but neglects customer experience and enterprise knowledge. Reimagine AI interactions with Reflected Intelligence. RI infuses AI with your organization’s DNA, fostering natural, engaging experiences that resonate with your brand. By understanding your company culture and communication style, RI builds trust and drives user adoption. Unleash the true potential of AI with Reflected Intelligence.
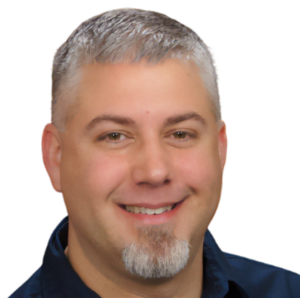
Principal AI Consultant, PTP
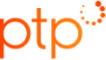
Chance has more than 25 years of experience working in the field of contact centers. My goal is to help my clients surpass their customers’ expectations and improve their operational benchmarks. By utilizing advanced technology, I focus on improving the customer experience and operational efficiency. This transforms the contact center ecosystem and makes it ready for the future.
[email protected]
PTP is a professional services firm delivering innovative customer service solutions across contact center infrastructure platforms that cut costs, enhance investments, and improve customer satisfaction.
Go Back to All Articles. Have a story idea? Submit to [email protected].
Want to get this publication in your inbox? Subscribe here!
The modern CX landscape is rapidly evolving, driven by the convergence of emerging technologies such as AI, advanced data analytics, and human emotional intelligence (EI). This “AI-Human Alliance” represents a transformative opportunity for organizations to deliver exceptional CX at scale — personalized, accurate, compassionate and profitable.
This is an interactive workshop where we’ll explore how to unlock the power of human interaction through technology to boost satisfaction, streamline processes, and empower employees to deliver faster, more personalized, and emotionally intelligent interactions.
Key takeaways:
- AI-powered strategies: Optimize processes, enhance customer experiences, and drive efficiency through the AI-Human alliance.
- Empowered workforce: Leverage AI to resolve complex issues, foster customer loyalty, and drive growth through predictive insights, personalization, and process automation.
- Scalable, agile operations: Design a CX model that balances technological innovation with human ingenuity for your organization’s competitive edge.
Join us for this hands-on session that will reshape how you think about AI, operations, and customer service—leaving you with actionable strategies to drive real impact.
Incorporating various technologies and working alongside outsourcers produces the best outcomes when alignments are in place and all parties are in sync. Without these synergies, brands risk inefficiencies across their operations. This session will explore how Ulta, in collaboration with IntouchCX, leverages innovation to shape its technology roadmap, enhance insights and analytics, and optimize workforce planning. The discussion will focus on how strategic sprints, integrated solutions and automation can drive efficiencies. This session will highlight real examples, showcasing how transparency, collaboration, and automated workflows have fostered positive change.
CX Leaders understand AI’s impact on helping customers, but there are important choices to navigate. Should you invest a lot of time and resources in custom-building a solution, or should you take an afternoon to launch it and help customers immediately? Speed to operation matters, and adopting AI that impacts CX doesn’t have to be a long, complex process.
Flip has powered ninety percent of successful Voice AI deployments by retail eCommerce brands. In this live session, you’ll get hands-on experience as Flip enables a retailer to quickly integrate and deploy Voice AI — in one afternoon. Witness instant automation and implementation, with customer experience improvements from Day 1.
Attendees will:
- Hear Flip’s Voice AI in action as it interacts with a caller and resolves a real-time support issue.
- Learn how Voice AI can enhance customer experience metrics while reducing costs.
- Discuss how Voice AI can transform your phone channel into a revenue-generating asset.
Traditional customer research captures moments in time, but customers experience brands over months and years. What if you could continuously capture authentic customer stories as they unfold, revealing hidden pain points and opportunities that surveys and focus groups miss?
This hands-on workshop introduces a transformative approach to customer understanding: creating a living library of real-world experiences that evolves alongside changing customer needs. Through video diaries, longitudinal tracking, and AI-powered analysis, organizations can move beyond point-in-time data to understand the Total Experience in real time.
Through interactive exercises and real-world examples, you’ll learn how to:
- Build rich video repositories capturing authentic customer interactions
- Identify emerging trends and friction points before they appear in traditional metrics
- Transform ethnographic insights into actionable CX improvements
- Use AI to analyze thousands of customer moments and surface meaningful patterns
- Design a continuous customer listening program that reveals unmet needs
- Create feedback loops that drive rapid CX optimization
- Build internal support for ethnographic research approaches
- Connect customer stories to business outcomes to drive revenue growth and retention
Workshop Outcomes:
- Tools for identifying key customer moments to capture and analyze
- Strategies for translating ethnographic insights into measurable CX improvements
- Methods for communicating the business impact of longitudinal research
- Templates for crafting compelling customer stories that drive organizational change
- An action plan for shifting from periodic to continuous customer research
Join us to explore how continuous ethnographic insights can transform your CX strategy and create deeper customer relationships.
The hype around generative AI’s (GenAI) potential to transform customer experience is real. And so is the potential for it to create meaningful lift and impact for businesses. The catch is that it’s not a one-size fits all approach and GenAI is most effective when housed within structured business processes. Join NLX Chief Customer Officer Christian Wagner and Field CTO Josh Schairbaum for a discussion that unpacks how GenAI can deliver the most value for enterprises.
We will explore strategies for how to apply GenAI to customer-facing use cases, strategies for identifying the best use cases to start with, and go under the hood to show attendees how to build a workflow that taps the GenAI within your structured business processes.
You wouldn’t use a hose to build a desk, just as you wouldn’t rely on a shark as an emotional support animal. Having the right tool for the job is crucial—so why do so many companies still rely on PDFs for their knowledge management system?
Join Atlanticus and livepro for an insightful discussion on how one company transitioned to a more effective Knowledge Management System and the transformative impact it had on their operations. We will walk you through their journey, including the challenges faced, key learnings, and measurable results. By the end of this session, you will gain actionable insights into how a premier Knowledge Management Solution can drive efficiency, enhance customer experience, and future-proof your organization.
Key Takeaways:
- Common pitfalls of ineffective knowledge management systems
- Why Atlanticus chose livepro to overcome these challenges
- The measurable impact of livepro’s implementation on efficiency and customer service
- Successes and challenges encountered during the transition
- How integrations with other tools amplified positive outcomes
- The surprising role of a good mascot
- The evolving relationship between AI and Knowledge Management and its impact on the future of customer service
Discover how Mosaicx and Bank OZK have partnered for over four years to continuously modernize their customer experience. In this mini case study session, you’ll learn how Bank OZK transitioned from a legacy IVR system to an AI-powered Intelligent Virtual Agent, now known to customers as Ozzy. Since implementation, Bank OZK has achieved significant improvements in customer interactions, boasting a +4% year-over-year increase in containment and consistently streamlining their operations for both general business and local branches.
Attendees will gain insights into:
- The strategies behind Bank OZK’s successful migration to AI-driven customer interactions.
- How end-to-end call analytics unlock deeper insights into customer interactions to drive greater automation and adoption as well as predictive intent within and beyond Bank OZK’s IVA.
- Real-world lessons on scaling AI capabilities for sustained growth and improved customer satisfaction.
Traditional Learning Management Systems (LMS) have been a cornerstone of employee training for years, but they often fail to keep pace with the fast-changing demands of today’s CX teams. Too often, these programs turn into check-the-box exercises, delivering little value and falling short of creating real impact.
In this session, Reddy’s CEO and Harte Hanks reveal how they reimagined CX training by developing a next-generation program built on AI-driven simulations, continuous learning, and applied skill development. By revamping the curriculum for a Fortune 500 client, they created a transformative solution that not only elevated agent performance but also delivered superior customer outcomes and a better overall agent experience.
Discover how this innovative approach redefined Harte Hanks’ training strategy—reducing ramp time, cutting average handle time (AHT), improving call quality, and empowering agents to excel in dynamic, high-pressure environments.
Quality assurance is an important part of every great CX strategy, but reviewing 100% of agent-customer conversations across every channel is an impossible task – until now.
It’s time to take the guesswork out of quality assurance and let AI do the heavy lifting. During this session, you’ll see practical, real-world examples of how automated QA reduces customer churn, saves time and resources, and pinpoints agent training needs, all while boosting service quality and customer satisfaction. You’ll leave with the knowledge and tools needed to leverage automation for a competitive edge, along with real-life stories and data to back it all up.
Key takeaways include:
- How automated QA tools improve the customer and agent experience
- Practical strategies for implementing automated QA
- A look at reporting tools to help you gain insights and review performance trends
- Case studies of organizations already realizing the benefits of automated QA
Discover how global powerhouses Google and Sony PlayStation are revolutionizing customer experience through GigCX in collaboration with Limitless. In this dynamic session, you’ll hear firsthand how these brands are harnessing the power of freelance brand ambassadors to drive unparalleled flexibility, scalability, and customer satisfaction. Gain exclusive insights into their successes, the obstacles they’ve overcome, and practical strategies for implementing GigCX in your own organization. Plus, engage in a live Q&A with industry leaders to get expert guidance tailored to your business needs. Don’t miss this opportunity to stay ahead in the evolving customer experience landscape!
AI is evolving fast, and CX leaders are under pressure to take action and deliver real results — all while navigating legacy systems, upskilling teams, addressing privacy concerns, and sifting through an overwhelming number of solutions to separate real value from hype. AI initiatives risk losing momentum without a clear, strategic roadmap before they create meaningful impact. In this interactive session, you’ll walk away with actionable strategies to define, fund, and execute an AI-powered CX strategy that drives tangible results in your business. By designing your own AI Success Roadmap aligned with your unique goals, you’ll be equipped to secure stakeholder buy-in and lead transformative CX outcomes.
Join Alvin Stokes, VP of Global Reservation and Service Operations at Princess Cruises, and harpin AI’s CEO, Scott Sahadi, as they reveal how a data-driven transformation reshaped Princess Cruises’ call center operations, improved guest experiences, and unlocked revenue opportunities.
Working together, they realized that prioritizing data quality and enhancing Princess’ data pipelines could significantly boost ROI—an often neglected challenge.
By improving data accuracy, integrating systems, and equipping agents with real-time insights and tools, they transformed these improvements into tangible results: reducing churn, lowering costs, and strengthening customer loyalty through personalized services.
Princess Cruises turned customer data quality from an overlooked challenge into a strategic advantage, enabling a successful omnichannel approach that delivered seamless service across customer touchpoints.
During this session, Alvin and Scott will discuss:
- How to fix fragmented data and start your customer data hydration journey.
- How to leverage observability and data science to investigate data quality issues.
- How to implement key initiatives that reduce costs and drive revenue growth.
- How to use data-driven insights to improve retention, reduce service costs, and enhance guest experiences.
- How to integrate disconnected systems and eliminate inefficiencies while maximizing existing investments in people, processes, and technology.
This transformation gave Princess the visibility it had been missing—turning an invisible problem into one they could track, understand, and resolve. Don’t miss this opportunity to hear from industry leaders about the strategies behind their success.
Join us for an insightful roundtable discussion on Turo’s AI adoption journey and the transformative impact on customer support operations. Jerry Howe, VP of Customer Support at Turo, and Dave Sheridan, VP of Sales & Marketing at Laivly, will share how Turo implemented AI using a structured “walk-run-fly” methodology—starting with AI-powered transcription and insights, scaling into real-time agent guidance, and laying the foundation for automation and quality assurance.
Discover how Turo leveraged AI to scale customer support while maintaining quality, improve agent decision-making, and streamline workflows. Learn how their phased approach enabled a smooth transition from AI-driven insights to automation, driving efficiency and customer satisfaction.
In this discussion, you’ll gain practical tips on:
- Walk – Using AI-powered transcription to capture conversation insights and enhance documentation
- Run – Implementing real-time agent guidance and automated case notes to improve decision-making
- Fly – Leveraging AI-driven automation and quality assurance to boost operational efficiency and customer experience
Designed for CX leaders, AI decision-makers, and contact center strategists, this session will provide actionable insights into simplifying AI adoption while maximizing its impact. Whether you’re just beginning your AI journey or looking to scale, you’ll leave with a roadmap for success.
Transform fragmented customer journeys into seamless experiences by starting with design and ending with technology, not the other way around.
For years, customer support organizations have navigated waves of technological change—from on-premise to cloud, from human agents to bots, and now the rise of AI. But too often, companies have taken a “lift-and-shift” approach, layering new tools onto old processes. The result? Fragmented experiences and mounting technical debt —frustrating both customers and stakeholders.
It’s time for a reset. In this session, COPC will introduce a proven framework for building AI-native customer journeys with purpose. Learn how to break free from outdated systems, align people and processes with technology, and create experiences that are intuitive, connected, and future-ready. Experts bring real-world examples to life, offering a clear path to designing seamless, AI-enabled customer experiences from day one.
In this discussion, you’ll learn how to:
- Design with intention—begin with the journey, not the tools.
- Eliminate legacy constraints with AI-powered solutions that reduce technical debt.
- Measure and refine customer experiences with a continuous improvement approach.
- Engage with peers in an open discussion on challenges, successes, and strategies for CX transformation.
- Apply a practical framework to align people, processes, and technology for end-to-end AI-native experiences.
This session is for CX leaders who are ready to move beyond incremental fixes and reimagine customer journeys with purpose and innovation.
An engaging session featuring real-world success stories from global leaders, Google and Sony PlayStation, in partnership with Limitless. This session will showcase how these iconic brands are leveraging GigCX, through empowering brand ambassadors, to deliver unmatched flexibility, scalability, and customer satisfaction. Attendees will gain exclusive insights into the results achieved, challenges overcome, and how to adopt GigCX. You will have the opportunity to engage in Q&A with industry leaders, gaining firsthand advice to apply to your own organization.
As customer experience evolves beyond digital transformation hype, organizations face complex decisions about where and how to invest in service excellence. This panel pulls together seasoned CX leaders to tackle pressing questions that directly impact business value and competitive advantage like:
- How are progressive leaders quantifying and communicating the true ROI of customer experience investments?
- Has the CX community become too focused on metrics at the expense of true customer advocacy? What capabilities are becoming more critical for service professionals in an AI-augmented environment?
Join members of the Execs In The Know Advisory Board, Lisa Oswald, Senior Vice President, Customer Service at Travelzoo; Pete Mallot, Worldwide Support Leader for Modern Life and Business Programs at Microsoft; Carolyne Truelove, Vice President, Reservations and Service Recovery at American Airlines, and Todd Sale, Senior Vice President, Customer Experience at Corpay, as they share their unique insights on where to invest, what to measure, and how to demonstrate CX’s direct contribution to business success.
Customer experience leaders often face a familiar challenge: gathering valuable insights through surveys but struggling to see those insights turned into meaningful, client-centric actions. Even when business partners express interest, the outcomes often miss the mark of truly serving the client’s needs. At KeyBank, the CX+EX team operates with a clear and ambitious mandate—to ensure clients’ voices are not just heard but reflected in every experience the brand delivers.
In this keynote, Era Ziroe, Senior Vice President, Enterprise Client & Employee Experience Director, KeyBank, will take you behind the scenes of KeyBank’s innovative approach to CX management. She’ll share how her team’s unique structure and collaborative methods are reshaping the way insights are actioned upon, ensuring client needs drive decision-making and business outcomes. Learn how KeyBank leverages empathy, insights, and proactive collaboration to meet—and exceed—client expectations.
Join Era to discover how KeyBank’s CX team’s model is setting a new standard for delivering both business success and exceptional customer experiences. Don’t miss this opportunity to gain actionable strategies to elevate your CX practice.
In today’s customer-driven market, companies are increasingly leveraging data to craft compelling Customer Experience (CX) stories that resonate with consumers. Businesses can gain deep insights into customer preferences, pain points, and expectations by harnessing data from various touchpoints—such as customer feedback, behavioral patterns, social media interactions, and transactional data. This data-driven approach allows companies to create personalized and engaging CX narratives that reflect their commitment to understanding and meeting customer needs.
Through advanced analytics, organizations can visualize the customer journey, identifying key moments that shape perceptions and influence loyalty. By analyzing quantitative metrics (e.g., Net Promoter Scores, Customer Satisfaction scores) and qualitative insights (e.g., customer reviews, sentiment analysis), businesses can illustrate how they have evolved in response to customer feedback, showcasing improvements and successes in delivering exceptional experiences. Data allows for continually refining CX strategies, enabling companies to highlight their responsiveness, adaptability, and focus on long-term customer relationships. Ultimately, using data to tell a CX story not only strengthens brand credibility but also fosters trust and emotional connections, setting companies apart in an increasingly competitive marketplace.
As AI automates routine tasks like FAQs and simple requests, customer service agents are increasingly vital for handling complex, high-emotion interactions that require empathy, critical thinking, and problem-solving. This shift demands hiring for emotional intelligence and communication skills, along with the ability to collaborate with AI tools.
Training must adapt to prepare agents for hybrid roles, where they enhance human interactions through AI support. As agents take on more specialized, high-impact tasks, compensation must reflect their growing importance as brand ambassadors. Organizations must rethink hiring, training, and retention to ensure agents are both technically and emotionally prepared to excel in an AI-enhanced landscape.
CX Livewire: Polling, Predictions, and Instant Feedback is an engaging 30-minute experience where the panel turns the spotlight on the audience! The panel will introduce a key topic and ask poll questions to gather real-time audience feedback. Panelists will predict audience responses and then dive into a lively discussion to explore whether their predictions were spot-on or missed the mark. This interactive format offers a unique way to gauge audience sentiment and spark conversation around real-time insights—perfect for CX Leaders looking to stay ahead of emerging trends.
Explore how Artificial Intelligence is transforming the landscape of customer experience, not just through efficiency, but by creating deeper, more meaningful interactions. In this panel, we’ll dive into how AI tools like chatbots, recommendation engines, and sentiment analysis systems are being used to enhance empathy and personalize customer engagement. Learn how businesses are leveraging AI to build emotionally intelligent connections with customers, driving satisfaction, loyalty, and long-term relationships. Discover the future of AI as a catalyst for delivering humanized, impactful customer experiences.
In 2023-2024, Spirit Airlines embarked on a major brand overhaul for Guest Care, creating the perfect opportunity to elevate its Guest experience. Vanessa Hardy-Bowen, Director of Guest Care & Contact Centers at Spirit, had already been leading efforts to integrate AI into the airline’s customer service operations. The brand transformation provided a pivotal moment to implement her vision of a cutting-edge, AI-powered contact center.
In this case study, Vanessa will walk you through Spirit Airlines’ AI journey—from identifying the right solution with her technical team to overcoming challenges like a failed AI voice pilot and addressing security concerns around generative AI. You’ll gain valuable insights into the airline’s approach to enhancing guest interactions and the results they’ve achieved so far.
Key takeaways for attendees:
- Strategies for aligning stakeholders and building a cross-functional team, including IT
- An overview of AI options for customer experience, along with their benefits and limitations
- Tips for managing stakeholders with differing priorities
- Factors to consider when choosing between a phased AI adoption or a full-scale implementation, including channel strategies
- Essential metrics to measure and understand AI agent performance
Join Vanessa to learn how Spirit Airlines is shaping the future of customer experience through AI.
In an era of rapid innovation and evolving customer expectations, transforming your organization to meet the needs of customers is critical. Join keynote speaker Christie Sandoval, Senior Vice President of Consumer Experience at T-Mobile US, as she shares how T-Mobile, one of the largest telecommunications companies in the U.S., is integrating the power of technology with human touch in their mission to deliver the best customer experiences possible.
With people at the heart of day-to-day customer interactions, Christie emphasizes the importance of empowering employees with AI technology and superpowers to ultimately uplevel and elevate the customer experience. This requires consistently assessing and anticipating customer needs and an inside-out, people-first approach to effectively operationalize change, foster a customer-obsessed culture, and collectively push the status quo.
Christie leads over 15,000 employees across the U.S. – the highest-ranked, most-awarded customer service team in the industry. She is deeply passionate about fuelling a culture where employees can be their most unique selves, grow personally and professionally, and do their best work. Her keynote will provide invaluable insights into how T-Mobile’s unique E2C (employee-to-customer) approach, powered by technology and data, translates to human-centric customer experiences.
In the dynamic world of hospitality, staying ahead requires unwavering dedication to continuous improvement and the embrace of a growth mindset. Join Frid Edmond, Senior Vice President of Global Customer Engagement Centers at Marriott International, in her transformative talk on “Leading Innovation and Change,” focusing on the strategies and leadership needed to foster innovation and growth.
In her keynote, Frid will share insights from her remarkable journey of leading teams through transformational change. She will reveal how she masterfully integrated loyalty programs after a large-scale merger, realigned organizational structures, and spearheaded technological advancements at a Fortune 500 company. Her insights will illuminate a range of innovative strategies and approaches, demonstrating how to navigate and implement these significant changes to achieve organizational success.
Attendees will gain invaluable insights into the leadership principles driving transformation. This session will spotlight the power of a “growing forward” mindset—encouraging continuous learning, adaptability, and proactive problem-solving to help individuals shift from resistance to resilience. Key themes include Courageous Leadership: making bold decisions and taking calculated risks; Persistence: overcoming obstacles and staying committed to long-term goals; and Resilience: adapting and thriving in the face of challenges.
Explore how these strategies can be applied to your organization, driving sustainable growth and positioning your business for long-term success. Whether you’re looking to enhance your organizational capabilities or lead your team through transformative change, this keynote will provide the inspiration and tools you need to thrive in an ever-changing market.
Travel has always been about people — but as technology and innovation reshape the journey, how do we ensure the human touch remains at its heart? Keynote Alvin Stokes, Vice President of Global Reservation and Service Operations Guest Services at Princess Cruises, shares his journey of transforming guest experiences through the power of data science and machine learning.
Learn how Princess Cruises overcame systemic challenges, improved data quality, and leveraged cutting-edge tools from Harpin and Salesforce to deliver contextually relevant, personalized interactions to guests. From wearable technology that elevates onboard experiences to agent-facing personalization tools that empower meaningful customer connections, Stokes will reveal the strategies behind the premium cruise brand’s 20% improvement in operational efficiency/budget and customer satisfaction.
Discover the untapped potential of data science, the importance of data quality in achieving personalization at scale, and real-world case study insights into creating seamless, always-on engagement. Whether it’s mapping the customer journey, fostering loyalty through data-driven insights, or balancing technology with human-centered service, this keynote explores the intersections of possibility that drive CX excellence.
As AI automates routine tasks like FAQs and simple requests, customer service agents are increasingly vital for handling complex, high-emotion interactions that require empathy, critical thinking, and problem-solving. This shift demands hiring for emotional intelligence and communication skills, along with the ability to collaborate with AI tools.
Training must adapt to prepare agents for hybrid roles, where they enhance human interactions through AI support. As agents take on more specialized, high-impact tasks, compensation must reflect their growing importance as brand ambassadors. Organizations must rethink hiring, training, and retention to ensure agents are both technically and emotionally prepared to excel in an AI-enhanced landscape.
Come see how the World’s Leading AI Simulation Coaching company has brought Generative AI to life for your frontline team members. You’ll see for yourself why they can’t get enough of their personal AI Coach.
It’s no mystery that repetitive practice produces consistent results. Providing that hands-on learning for both new hires and tenured associates at scale without technology is unreasonably expensive, inefficient, and nearly impossible. Zenarate’s AI Coach allows companies worldwide to overcome each of these challenges.
If you want to learn how leading brands have delivered the following results with AI Coaching…
- Improved speed to proficiency by 70%
- Reduced attrition by 52%
- Cut training expenses by 50%
- Improved conversion rates by 34%
- Increased QA + CSAT by 47%
…then don’t miss this interactive innovation session delivered by Learning & Development expert Casey Denby.
Come experience AI Coach for yourself.
You can have both – improved CSAT and AI automatically answering support requests.
Discover how one of our clients is doing just that and how they:
- Gained leadership and team buy-in for AI-first customer service
- Prepped for and launched an AI Agent
- Optimize AI agent performance for their organization
- Improved CSAT at the same time
Whether you’re still preparing to adopt AI for customer support or want to improve your resolution rates, this session will provide you with specific tactics and concrete advice to successfully incorporate AI into your strategy.
Unlock the power of employee engagement to drive exceptional customer experiences
in this interactive 1.5-hour workshop. This session explores the fundamental principles
of engagement and their practical application in the workplace.
Participants will:
- Dive deep into the core tenets of employee engagement
- Understand how the Great Place to Work (GPTW) survey aligns with
engagement principles - Analyze real-world examples of engagement initiatives tied to GPTW categories
- Develop actionable plans to address common engagement challenges
- Learn from OP360’s successful engagement strategies
Through group discussions, scenario-based problem-solving, and shared experiences,
attendees will gain insights into:
- Creating a positive work environment that enhances employee satisfaction
- Improving customer service outcomes through engaged employees
- Implementing effective communication, recognition, and growth opportunities
- Fostering fairness, purpose, and autonomy in the workplace
Leave with a toolkit of practical strategies to boost engagement, including:
- Flexible scheduling for work-life balance
- Recognition programs that motivate and inspire
- Professional development initiatives
- Wellness and inclusion programs
Join us to learn how to nurture a thriving workplace culture that benefits employees,
customers, and your bottom line.
Join us for an insightful roundtable discussion on the latest AI-powered tools revolutionizing the contact center landscape. We’ll explore cutting-edge technologies with a leading luxury retailer including agent assist, automated summarized notes, fraud detection, auto reships and automated email responses. This session is designed for CX leaders eager to enhance efficiency, accuracy, and security in customer interactions. Attendees will gain actionable insights into how these tools can streamline operations and elevate customer satisfaction.
Discover how AI can provide real-time support to agents, offering suggestions, answers, and guidance during customer interactions to improve response times and accuracy. Understand the role of AI in detecting and preventing fraudulent activities during customer interactions, safeguarding your business and customers and more!
In this discussion, you’ll gain practical tips on:
- Implementing agent assist tools to enhance real-time decision-making during customer calls
- Utilizing automated note summaries to improve agent productivity and reduce after-call work
- Integrating fraud detection systems to protect your contact center from security breaches
- Adopting auto reship capabilities to streamline the replacement process and reduce customer frustration
- Setting up automated email responses that maintain personalization while increasing efficiency
In today’s dynamic business environment, organizations increasingly recognize the importance of effective support models and strategic partnerships to enhance operational efficiency and customer satisfaction.
This panel explores developing and implementing robust people and agent support systems, emphasizing the significance of well-designed partnerships between businesses and their support teams. Whether your organization leverages outsourced services or manages operations internally, navigating agent support requires strategic insight and adaptability.
Support models must stand ready to solve complex problems, drive digital transformation, and leverage technology to elevate the customer and employee experience. By fostering collaboration and leveraging the strengths of both internal and external resources, companies can build a support infrastructure that is both responsive and resilient.
This Panel Discussion will provide valuable insights into:
- Best practices for creating and maintaining partnerships that align with organizational goals.
- The value of strong comprehensive support models that can meet the evolving needs of businesses and their customers.
- Leveraging the tools and technology that can strengthen your agent delivery model.
- Join this conversation to learn how to navigate agent support and ensure better client, customer, and employee experiences.
You’ve implemented automated solutions but how well are they being operationalized to drive measurable results? Automation is impacting all facets of business and getting the most out of it requires experience and insights to successfully integrate it across your entire CX lifecycle.
Join Matt Wheatley, Chief Revenue Officer at IntouchCX and Ingrid Betts, SVP of Transformation and Strategy at Conservice for a deep dive on strategic automation integration and how it can impact the bottom line.
Key Takeaways:
- How to integrate AI & automation across your CX operations
- Identifying & enabling automation across the customer & agent journey
- Leveraging expertise in platform and tech stack integration
Delivering exceptional customer experiences (CX) requires more than just implementing the latest technologies. It demands a holistic, long-term approach that transforms organizational culture and mindsets.
This session will examine strategies for creating a sustainable culture of CX transformation, where continuous improvement, agility, and customer-centricity become embedded in the enterprise’s DNA.
It will explore the key elements needed to drive lasting change, including:
- Leadership alignment
- Employee engagement
- Process optimization
- Data-driven decision-making to drive business value
In this case study presentation, we delve into the successful Mastercard CX journey, showcasing how they have transformed CX through a human-centered approach.
Key discussion points include:
- Mastercard’s digital transformation journey and the role of partners like TELUS International in supporting their vision.
- The importance of mastering foundational CX operations before implementing AI and other digital technologies.
- How Mastercard and TELUS International have balanced human and AI collaboration to enhance customer service.
- Specific examples of successful CX transformation.
- Insights and advice for CX leaders on leveraging AI – what it can and cannot do
Customer service leaders are under constant pressure to drive growth and customer satisfaction with innovative solutions. In this panel led by Freshworks, CX industry leaders will share critical insights and considerations they face when looking to drive rapid innovation in their organizations. They will discuss the critical foundations they focus on to ensure their innovation decisions drive the business results they seek and are future-proof.
You will gain insights into:
- The power of data in ensuring a unified view of your customer
- Embracing AI to drive scale, trust, and security
- Enabling key leader insights to drive accurate decision-making
Through engaging discussions and hands-on exercises, you will develop a clear understanding of the essential platform capabilities required to drive exceptional customer service and achieve specific business objectives. Sharpen your platform selection skills through interactive exercises designed to evaluate key capabilities and ROI, including:
- Developing a blueprint to unify customer data
- Creating a roadmap for AI deployment
- Identifying proactive, real-time KPIs
Don’t miss this opportunity to network with industry peers and gain actionable strategies to propel your organization forward.
The goal of this panel session is to explore how empathy and emotional intelligence (EI) can serve as transformative forces in customer experience (CX) management, transcending traditional metrics like Net Promoter Score (NPS) and Customer Satisfaction (CSAT) scores. In an era where customers expect more personalized and meaningful interactions, the integration of empathy and EI into CX strategies is becoming increasingly vital.
This session will feature a panel of subject matter experts (SMEs) who will share insights and real-world examples of how leading organizations are incorporating these human-centric approaches to enhance customer loyalty and satisfaction. Through interactive discussions, we’ll lean into challenges and opportunities associated with fostering an empathetic culture within CX teams and company culture.
CX leaders will leave with actionable ideas, compelling anecdotes, and valuable resources to guide the adoption of empathy-driven approaches in their own organizations. This session aims to inspire a shift from viewing customer interactions as mere transactions to embracing them as opportunities to build deep, emotional connections that drive long-term success.
As AI transforms the contact center, supporting people managers becomes critical. During this session, we’ll dive into empowering managers to thrive in this new era.
Join our session to learn how to:
- Foster decision-making and problem-solving by managers.
- Promote continuous learning for effective leadership.
- Leverage AI tools for data-driven coaching and personalized experiences.
- Balance automation with the human touch for empathy and success.
Through practical examples and interactive discussions, participants will gain actionable insights into creating an environment where agents and managers feel empowered, supported, and equipped to succeed in this new era of AI-driven contact centers.
In today’s fast-paced digital landscape, delivering exceptional customer experiences is more critical than ever. Join us for an in-depth session where we explore how Limitless, in collaboration with Google, has harnessed the power of GigCX to transform the way customer support is delivered.
In this session, we will delve into the innovative strategies that have placed customers at the heart of the customer experience, leveraging the flexibility and scalability of GigCX. You’ll discover how Limitless and Google have reimagined traditional customer service models, empowering gig experts to deliver personalized, empathetic support at scale, and in real-time.
This case study will provide actionable insights into how your organization can adopt similar approaches to revolutionize customer engagement, drive customer satisfaction, and ultimately, foster deeper brand loyalty by putting the customer first in every interaction. Don’t miss this opportunity to learn from industry leaders and take your customer experience strategy to the next level.
Take a journey with leading brands demonstrating significant results in this large language model (LLM) era. While most Generative AI (GenAI) RAG options are getting caught up in legal and compliance issues, discover how some of our favorite brands are accomplishing customer self-service rates with modern virtual assistants.
Many experts are ignoring another option that delivers tremendous results. Explore how Fortune 500 consumer brands use LLMs for speed, ease, and scalability without risks. In this Tech Forum, we will deep-dive into real customer-facing results that compare and contrast to legacy virtual assistants that will soon become extinct.
Staples aims to help businesses of all sizes be more productive, connected, and inspired. With the introduction of generative AI (GenAI), Staples quickly recognized its potential to enhance the experience of store employees (Associates) by improving their ability to serve in-store customers and increasing their confidence, productivity, and job satisfaction. The company sought a partner to bring their vision for a conversational AI assistant capable of addressing both Associate and customer queries to life and found that partner in Quiq.
Since deploying its employee-facing GenAI Assistant, Scout, Staples has streamlined and accelerated access to information. Scout, an AI model trained on all things Staples, can instantly answer questions about policies, processes, products, and more, enabling Associates to assist customers and themselves efficiently.
Join this discussion with Natasha Bialecki, Staples CX Specialist, and Quiq Founder and CEO Mike Myer to learn:
- Key considerations when developing a GenAI Assistant
- Unexpected challenges encountered during the implementation of Staples’ first GenAI Assistant
- Strategies for scaling a GenAI Assistant rollout, including innovative approaches to drive Associate usage and engagement
When employees don’t feel valued or motivated to do meaningful work, the negative impacts are deep and wide. But where should leaders focus to make sustainable change? This workshop cuts to the core of employee apathy and arms leaders with actions that transform the perception of contact center work.
- Participants will explore:
- What are the root causes of employee apathy and how do you address the early indicators?
- Which methods are best for understanding employee sentiment, motivators, and detractors?
- How does clarifying and strengthening the connection between employee purpose and business goals improve performance?
Astound Communications, an award winning internet service and 6th largest telecommunications company in the United States, faced common challenges in selecting and implementing an AI solution to enhance their contact center operations. Their objectives for AI were to increase productivity, lower attrition, reduce training costs, and enhance agent efficiency. Ultimately, Astound choose Agent X, deploying an LLM-driven QA solution.
In this discussion, HGS and Astounds Communications share a journey of understanding, evaluating, selecting, and implementing an AI-powered knowledge management solution. Learn how the implementation led to a comprehensive dashboard that boosted agent productivity, allowed for the leverage deeper insights, and offered a 360-degree of the customer to enhance the end user experience.
Gain key insights into:
- Criteria for Selection: Understand the specific criteria Astound Communications used to identify an AI solution that aligned with their goals.
- Cost Efficiency Gains: Find out in what ways an AI-based system can drive cost efficiencies.
- AI Implementation: Learn about what success looks and how to measure it when deploying of an AI-based intelligent knowledge management solution.
- Automated QA Solution: Explore how the integration of an LLM-driven automated QA solution further optimized operations.
- Agent X Solution: Discover why Astound Communications selected Agent X as their AI-powered contact center solution
In an era of rapid disruption in traditional car retail and financing, Chase Auto, a division of JPMorgan Chase, is leading the transformation of car buying and vehicle financing. Join keynote speaker Renée Horne, Chief Marketing & Customer Experience Officer, as she shares how Chase Auto is pioneering a shift towards innovative, customer-centric solutions that meet the evolving market demands.
Renée attributes her career success to being curious and a bit adventurous, in her keynote, she will discuss how passion for innovation allows Chase Auto to create a seamless, omni-channel car buying experience using advanced technologies and JPMorgan Chase’s extensive assets.
For manufacturers, Chase provides essential capital, financing, extensive marketing capabilities, customer data, an expansive branch network, and commerce solutions. For dealers, Chase Auto offers flexible credit options, alternative deal structures, and comprehensive banking services, transforming from a retail lender to a commercial banking partner. For consumers, Chase Auto revolutionizes the car buying journey with digital originations, pre-approved loan programs, and enhanced user experiences.
Renée will explain Chase Auto’s holistic approach to improving the automotive supply chain, providing reliable capital and services to manufacturers, financial solutions to dealers, and a seamless car-buying journey to consumers.
Join us to learn more about Chase Auto as they enable manufacturers to innovate, dealers to thrive, and consumers to achieve their vehicle ownership dreams, ultimately transforming the automotive industry.
Generative AI is the hottest topic in CX today, and one of its most promising applications is powering GenerativeAgent. A generative AI-powered virtual agent is as capable as Tier 1 agents to increase resolution and customer satisfaction. Your customers need a virtual agent that they can speak to naturally to accomplish tasks. Now they have one.
In this session, ASAPP and PTP will deep dive into this AI-led innovation and share how they partner with clients to deploy GenerativeAgent to deliver operational improvements, increased customer satisfaction, and reduced costs. You will witness firsthand just how powerful this technology is!
You’ll gain insights into:
- Ways GenerativeAgent unlocks business value and improve CX
- Real-world examples of how the ASAPP/PTP team is deploying GenerativeAgent with a Fortune 100 client and the results they are seeing
- Practical tips to get started on your organization’s GenerativeAgent journey, including best practices and process recommendations
The world is full of famous duos (Holmes & Watson, Batman & Robin, Han & Chewie), showing us that working together is better than going alone. The same can be said for the complex and ever-changing world of customer service. How can you be expected to stay up to date with the latest CX best practices when Generative AI is changing the game, sometimes by the hour? You need an experienced and innovative partner to navigate this ever-changing world and empower you to create craveable customer experiences.
By attending this session, you will learn:
- How Progressive identified the right partner to entrust with their customers
- How Progressive saves over $20M in annual operating expenses
- How Progressive achieves 97% intent accuracy to empower customers”
Mosaicx is a cloud-based solution that uses conversational AI, machine learning and natural language processing technologies to automate interactions with customers and employees. Its IVA technology delivers fast, easy, personalized service through industry-leading voice recognition and digital messaging capabilities, creating positive interactions that drive improved customer and employee satisfaction. Mosaicx makes conversational AI surprisingly simple.
AI in customer service is here. While some brands are still considering how it fits into their CX strategy, others are already hard at work implementing it in their day-to-day to handle volume, streamline processes, and grow customer loyalty.
In this panel led by Gladly, hear practical strategies and personal experiences from leaders on how they’ve applied AI across their support teams and found success.
They’ll discuss how to use AI to:
- Manage volume with intelligent solutions that meet every business need, from powerful self-service features to effective automation tools for agents.
- Scale and meet changing contact center needs, such as seasonal spikes in call volume, without increasing costs.
- Empower your agents to focus on high-value interactions — such as cross-selling and upselling — that drive revenue and customer satisfaction.
In an era where customer expectations continually evolve, transforming your organization to meet and exceed these demands is imperative. Join keynote speaker Kimberly Masters, Senior Group Director at Sam’s Club, as she unveils the journey of transformation that led to significant improvements in customer satisfaction and operational efficiency.
Kimberly emphasizes that a successful transformation starts and ends with an obsessive focus on enhancing experiences for members, customers, and associates. Achieving this requires a unified vision and measures of success across all levels, both internally and outsourced. Embracing boldness and the courage to challenge the status quo, coupled with integrating process, technology, and resources, is crucial. Timing is everything, especially concerning GenAI, and is essential for getting the experience right.
Her keynote will provide invaluable insights and actionable strategies for organizations looking to embark on their path to transformation, emphasizing the importance of a strong foundation, strategic alignment, and a bold, innovative vision.
Amanda Wiltshire-Craine, Senior Vice President and Head of Global Customer Services at PayPal, is a forward-thinking leader committed to personalizing customer experiences through technology and innovation. In her keynote address, she will discuss PayPal’s approach to customer service under the guidance of their new CEO and the brand’s ambitious three-year strategic plan to radically transform service delivery and operations.
With a focus on personalization and automation, PayPal is setting new standards for customer interactions. It is leveraging data and advanced technologies like AI and GenAI to become more predictive in how the organization surprises and delights its customers. Amanda will illuminate the journey toward completely reimagining servicing as more intuitive, empathetic, and universally accessible.
She is deeply passionate about diversity, equity, and inclusion as a leader, particularly in promoting leadership opportunities for women and people of color. Amanda aims to leave a legacy that empowers the next generation of leaders, making meaningful progress toward a more inclusive C-suite landscape.
Join Amanda to explore how PayPal’s innovative approach to service transformation can inspire your strategies for integrating technology with human-centric customer interactions.
Join us for an enlightening keynote with Emily Sarver, Vice President of Customer Experience at Lovevery, a subscription-based early learning company focused on child development. The brand, known for its dedication to social and environmental impact, has over 350,000 active subscribers and was named one of Fast Company’s Most Innovative Companies in 2024.
Emily is an intentional, dynamic, and forward-thinking leader passionate about building and scaling world-class support teams. She leads from a place of conviction, characterized by a commitment to continuous improvement and a clear vision for the future. During her keynote, she will delve into the CX strategies that have fueled Lovevery’s growth and innovation, including using every interaction as an opportunity to build a meaningful relationship.
You’ll gain an understanding of how acting for the good of society and the planet and having purpose-driven leadership, along with a people-first culture, can help scale operations globally and advance a brand’s reputation while maintaining a solid customer-centric focus.
Attendees will walk away with actionable insights and be inspired to implement purpose-driven leadership within their own organizations to achieve operational success and make a meaningful impact.
Lead your contact center into a future where AI and human agents work together seamlessly. COPC will provide a comprehensive strategy that addresses the technical and emotional aspects of AI adoption.
Using the COPC AI Impact Change Planning tool, participants will gain practical strategies to analyze the impact of AI on agents and create a plan to navigate AI integration. Leaders will learn methodologies that ease the transition, improve agent satisfaction, and increase adoption.
Facilitators will begin by discussing how AI is changing the agent role, followed by an interactive session to methodically analyze the impact of AI in critical areas such as:
- Agent skills and knowledge requirements
- Recruitment and training
- Agent metrics and performance management
- Staffing requirements
- Agent perceptions of AI
This session will culminate in a discussion that brings this information to life to facilitate agent adoption. Leveraging the COPC AI Impact Change Planning tool, you will walk away with a strategy that transforms agent experiences with AI, making their jobs better and more fulfilling.
Strategic outsourcing can transform businesses by providing unmatched scalability and access to global talent and deep industry expertise. Despite its advantages, misconceptions like loss of control, quality decline, and remote team issues deter companies.
Driven by operational and financial constraints, Public Storage collaborated with OP360 to explore offshoring as a strategy for achieving customer experience and operational excellence.
This fireside chat will dive into the hurdles, challenges, and ultimately benefits that come with offshoring through the eyes of first-time outsourcer Public Storage. We will unbox their journey including:
- The driving force behind the outsourcing decision.
- The partner model and strategic approach.
- Achieved outcomes compared to initial expectations.
Join us for a workshop focused on how modern AI tools and Analytics are helping enterprises big and small establish the operational building blocks designed to breed a culture of trust and excellence. Learn about ways to distill and protect your data, while also being able to leverage that data across your operations to effectively coach and mentor your frontline, measure the effectiveness of your coaching process, and build a healthy and committed culture of continuous improvement at all levels.
Every business speaks to customer satisfaction and values what a CSAT measure can tell them, but the most significant contributor to customer loyalty sits inside of your own organization. Your corporate culture dictates your customers’ satisfaction. What happens inside of your own four walls is as important as what occurs outside of them. Hear from the President and CEO of one of the nation’s most respected brands on how he fosters culture to drive satisfaction.
Philip Walker is the President and CEO of AAA Life Insurance Company, the life insurance business supporting the vast AAA membership – one of our nation’s most recognized membership organizations with over 60 million members.
Philip is a senior executive with 30 years of experience building and growing strong financial services companies. He will share how he delivers value to AAA’s membership base by understanding their needs and inspiring his team to develop a service-mindset. His vision to foster this corporate culture to serve the AAA member has grown the life insurance business and created loyalty within the overall AAA membership.
Join this live, power-packed session to see how AI-powered learning technology’s power has transformed the contact center training landscape forever. Training expert Casey Denby will deep dive into proven learning theory that has been incorporated into modern-day AI-based technology to scale learning efficiently and cost-effectively.
You don’t want to miss this session if you want confident, prepared, happy agents! Casey will share how leading brands such as United Healthcare, Bank of America, Verizon, Capital One, Prudential, Airbnb, Comcast, and many more have transformed their frontline training programs and found success via AI-Powered Simulation Training. Create the most lifelike, unbiased roleplay experience for your agents: it’s fast, flexible, and scalable.
In this session, you will:
Learn exactly what customers want from your brand and how you can best prepare your frontline agents to deliver through:
- Hands-on AI-Powered Simulation Training
- Understanding of ‘how people truly learn’
- Proven learning strategies you can take home and implement right away
- A live simulation build and practice that you can get your hands on and experience for yourself
Experience first-hand the potency of AI-Powered Simulation Training, as Casey shares:
- How to build a real-life simulation live
- Where GenAI is leveraged in the build
- A real simulated call scenario that you can practice live
In the dynamic world of technology, conversational automation has emerged as a groundbreaking tool, captivating industries with its potential to create measurable positive outcomes in customer engagement. This Tech Forum session offers a unique opportunity to delve into the success story of Home Depot, a trailblazer in redefining customer experience through the adept use of Large Language Models (LLMs) in a manner that is both safe and compliant.
We will explore the intricate distinctions between Retrieval Augmented Generation (RAG) and LLMs, drawing valuable lessons from Home Depot’s journey. Their approach exemplifies how conversational automation demands more than just guidelines and guardrails; it requires a strategic, transformative mindset crucial for achieving success in this domain.
Key takeaways will include:
- Confidence in Automation: Understand how embracing conversational automation in customer service can lead to significant benefits, offering a high return on investment. This segment will showcase concrete examples from Home Depot’s journey, highlighting key outcomes around containment and call resolution rates.
- Practical Roadmap: Gain insights into initiating and navigating the journey of conversational automation. We will dissect the essential considerations and steps necessary to implement this technology effectively, drawing from real-world experiences and the successes and challenges encountered by Home Depot.
- Understanding the Urgency to gain better Outcomes: Grasp the potential repercussions of delaying the adoption of conversational automation. The session will emphasize the urgency of integrating this technology to stay competitive and relevant in the rapidly evolving tech landscape.
- AI Fundamentals: Learn key differences between Retrieval Augmented Generation (RAG) and LLMs and why they matter to you as a leader.
This enlightening keynote address will dive into the transformative impact of customer-centric strategies on business success. In this engaging talk, Tracy Sedlak, VP of Customer Success at Offerpad, unravels the pivotal role of harnessing Voice of the Customer data in shaping robust business strategies, fostering a culture of customer-centric excellence, and embracing the power of stepping outside our comfort zones to glean unparalleled insights from customers.
Discover how these invaluable insights are not just indicators but catalysts for innovation and growth, driving organizations toward a future where understanding and leveraging the customer’s voice are at the heart of every strategic decision.
You can hear more about Tracy’s brand story and leadership journey at CRS Tucson.
The panel discussion delves into the intricacies of launching and sustaining AI-driven customer experience (CX) initiatives. The conversation will explore the landscape of AI implementation, emphasizing the elements necessary for successful deployment and continual support. Hear from Brands that have launched their AI strategies on what challenges they faced, the strategies they used, and the vital components they have planned to nurture and evolve their AI-based CX solutions beyond their inception. Get answers to real questions!
- What skills or roles are needed to stand AI up?
- What skills or roles are needed to sustain it?
- Where does AI for CX sit in the enterprise? Customer Service, IT, Product Management, or a brand new sub-team?
In the fast-paced world of customer support, where quick responses and resolutions are crucial, the arrival of AI has been nothing short of revolutionary. Among the champions leading the charge in AI-driven customer support, AppFolio stands out. AppFolio is an Intercom customer that provides software applications and services for the real estate industry.
This session will dive into how innovative companies like AppFolio structure their customer service teams in an AI-first future. In particular, we’ll uncover how Appfolio was able to adopt this new technology and achieve substantive results quickly, including:
- Increasing their self-serve rate and automatically resolving 30% of customer inquiries using AI
- Decreasing overall response times and time to resolution
- Increasing trial conversions through proactive support
Prioritizing enhanced consumer experiences is paramount for any consumer-facing brand. By integrating AI and automation, businesses can ensure timely and thoughtful responses to online consumers, freeing up resources to focus on efficiency, quality, and meaningful human interactions.
Join Kim McMiller, SVP Global Client Services at IntouchCX, and Luc Antoine, Head of Care at L’Oréal Canada, as they delve into how to harness automation for data integrity, aligning seamlessly with a global vision and deep understanding of consumer needs. This engaging workshop will highlight real examples from a top consumer brand and offer the unique opportunity to collaborate amongst your peers on leveraging automation, people, and processes to optimize operational efficiency and enhance the consumer journey.
AI is taking center stage when it comes to elevating customer service experiences. When we mix the smart capabilities of AI with the kind of empathy only humans can provide – that’s where the magic happens for both companies and their customers.
Our workshop will explore strategies combining the best technology with genuine, empathetic customer service to create experiences that truly differentiate and impact customer loyalty. Attendees will gain practical tips for prioritizing customer needs and emotions, ensuring seamless, personalized service that bridges the gap between digital efficiency and the human touch.
Sentiment… Language… Speech… QA… We all reference these terms, but do we know how to place tangible value on the human voice expressing such communication?
With the numerous human-based metrics used to manage corporate performance, most contact center operations have focused their investment decisions on advancements that remove the human component.
Yet, measures involving VoC, Promoters, and QA remain prevalent throughout all organizations. Many executives have sought to learn the true ROI involving sentiment… a high QA score… or even Top Box satisfaction. For many, attaching an ROI to these metrics appears daunting, moving them to a ‘necessary evil’ expense line. In the age of AI, we find ourselves pushing for investment success associated with corporate efficiency and reduced headcount, but how about the same rigor toward retaining humanity in our enterprises? Societal research continues to show that people resonate with and need personal connection. The relationship between our customers and brands should capitalize on that need while continuing to foster satisfaction with product offerings.
Hear from industry-leading executives who have capitalized on humanity’s power and created tangible business results. Then let’s collaborate as a Community to define what tangible success means for our Humanity Factors. Facilitated break-out tables will focus on customer sentiment, agent behavioral factors, customer satisfaction, and the difference between proactive and reactive communication analyses to design the next generation of ROI models as we embrace the AI era.
Dive deep into the future of financial services with Veronica Semler, Vice President of Servicing Operations at Oportun, as she demystifies the complex interplay between Generative AI and big data in revolutionizing the industry. Oportun, a distinguished FinTech provider committed to making financial goals achievable for over 2 million members, harnesses cutting-edge AI to reimagine customer service dynamics and operational efficiency.
This session promises a thorough dissection of AI-driven strategies reshaping how data is interpreted, consumed, and leveraged for decision-making. Witness firsthand how Oportun’s leaders, supervisors, and agents integrate these advancements to foster a culture of innovation, significantly enhancing customer experiences and driving financial success.
You’ll gain insights into:
- How consumable data eliminates the need for context switching and revolutionizes coaching and QA practices
- Real-world examples of how actionable insights derived from AI analytics empower staff at all levels to make informed, strategic decisions.
- The ethical considerations and governance of AI in managing sensitive financial data.
Consumer expectations for customer service are higher than ever before – they expect to engage with businesses across multiple digital channels that are convenient, fast, and efficient. Join Amazon Web Services (AWS) to discover best practices for how to leverage phone, in-app and web calling, video, chat, short message service (SMS), and third-party messaging to deliver exceptional customer experiences.
In this session, you will learn about:
- Executing a true omnichannel strategy that delivers consistent customer experiences and better outcomes
- Empowering customers with self-service solutions for increased speed of service
- Driving high-velocity innovation in your contact center to improve your customer experience
Navigating Moments of Truth: A Human-Centric Approach to Employee Engagement and CX Excellence
Join Intradiem’s President and COO, Jennifer Lee, as she leads a panel of industry experts in a discussion that extends beyond the traditional focus to explore the heart of employee engagement, uncovering genuine “Moments of Truth” for both employees and the brand.
As technology continues to impact the type and complexity of live calls, employee experience must remain a focal point. CX leaders must reexamine the agent’s role and establish targeted, effective employee engagement support mechanisms to ensure agents are best equipped to deliver exceptional “Moments of Truth” in these new modern customer interactions.
The conversation will navigate the intersections between these pivotal moments, revealing how mutual support shapes a powerful synergy. Discover how these moments profoundly impact CX and explore strategies for combining technology and acknowledging the human touch to positively influence your “Moments of Truth.”
Key panel takeaways will include:
1. How to find and define your “Moments of Truth”
2. The mechanisms needed to modernize and streamline agent workflow
3. How to put training at the center of your employee experience and engagement
4. Taking a proactive approach with technology to enable a better EX
Are you wondering how to incorporate AI and Automation into your program effectively? Need help figuring out where to begin or how to create a compelling business case? Join Laivly along with Abercrombie & Fitch as they share insights on navigating the new landscape of AI technology in CX. Learn how to fund your tech projects based on cost savings and ROI from the first interaction.
Discover how to identify that crucial first step and develop a persuasive investment story. Learn from real-world experiences where an unexpected, high-value, compliance-based use case was the foundation for broader AI and Automation implementation.
In this discussion, you’ll gain practical tips on:
- Finding early ROI opportunities
- Crafting a smart expansion plan
- Future-proofing your AI strategy
Join us and embark on the path to AI and Automation success!
Today’s service delivery landscape largely focuses on technology-driven solutions thanks to emerging channels, cutting-edge tools, and AI-powered insights. But without a human-centric approach, you risk losing the emotional connection and empathy needed to unlock the value behind your investments.
Real transformation requires a human-centered design to succeed, so ensuring your service delivery approach is modeled around (and for) people is crucial to delivering outstanding customer experiences. In this session, Tadd McAnally, VP of CX Advisory services at VXI, will discuss prioritizing the human element by applying design-thinking principles backed by real-world examples and success stories in an increasingly complex ecosystem.
Join this compelling Customer Shop Talk to learn:
- How to assess your service delivery model and its maturity level
- How to ensure your transformation roadmap is collaborative, agile, and human-centric
- How to synchronize your entire service delivery network around your transformation efforts
Don’t miss out on this important session — or the opportunity to design with your people (not just bots) in mind.
Dive into the cutting-edge of AI and revolutionize your customer experience (CX). Whether you’re just starting or already on the path, this is your invitation to a game-changing dialogue on AI’s role in elevating both customer and agent interactions.
Discover the stark contrast between legacy architectures and the dynamic future promised by AI-infused technologies. Learn about AI’s power to seamlessly assist with post-call enhancement, provide next-best action recommendations, IVR and dialog flow customization, and enrich your knowledge base.
Join us to explore how wrapping AI around your current IT infrastructure can drive intelligence, foster innovation, and inject agility into your operations. This is more than a chance to upgrade your technology—it’s a pathway to reshaping your organizational landscape and redefining the CX you deliver.
A customer doesn’t think about channels. Some communicate only on one channel; some effortlessly flit across channels. But brands and their Customer Experience (CX) leaders don’t have that luxury. They must manage preferences across all their customers while trying to strike the balance of service excellence, operational costs, and an array of automation and artificial intelligence (AI) capabilities across channels.
How do brands manage the tension between serving customers however they want, in whatever channel they want, and proactively steering customers to channels to manage customer experience and efficiency?
This panel will dissect modern frameworks for crafting effective customer channel journeys. We’ll delve into customer behaviors across channels and the importance of fostering lifelong conversations that don’t just span channels but span over the entire customer relationship, moving beyond mere transactions. Insights from diverse brands will shed light on the evolving strategies and critical decisions in the era of customer experience AI and automation.
Join us as we explore the seamless channel experience. Our focus will be on how brands can balance operational efficiency and customer-centricity in the digital age, underscoring the need to delight customers, build meaningful connections, and drive lifelong customer loyalty in the constantly evolving customer service landscape.
It’s often assumed that a decline in customer experience pays for gains in operational efficiency. That’s not the case for Bob’s Discount Furniture. They are leaders in both operational efficiency and NPS.
A customer-obsessed culture and great product value are the foundation of Bob’s brand. But more importantly, Bob’s also leverages digital channels and Generative AI at strategic points in their customer journey, allowing them to minimize phone contacts while still getting customers the information they need. It’s a win-win, offering great CX while minimizing costs.
Join a conversation with Quiq and Bob’s to learn:
• Insights into the techniques that Bob’s used to drive CX efficiency and the outcomes they achieved
• Understanding of the Generative AI Maturation Curve that Bob’s is using to plan the adoption of safe Generative AI
• Suggestions about how to navigate organizational challenges, risks, and barriers to AI “
Even the most reputable companies strive for constant improvement in today’s fiercely competitive business landscape. As technology reshapes traditional operations, it has become imperative for organizations to foster consistency, increase efficiencies, and create a high-performance culture. Join us for an insightful session featuring Lance Gruner, Mastercard’s Executive Vice President of Global Customer Care, and Kyle Kennedy, President and CEO of COPC Inc. They will reveal how Mastercard’s commitment to excellence led them to adopt and implement a unified performance management framework. Lance will share Mastercard’s impressive journey and its sustained impact on the organization and its customers. Don’t miss out on the chance to hear Mastercard’s story of continued success in the face of rapid change, solidifying its leadership position in the CX industry.
In today’s tech landscape, the promise of conversational automation has captivated industries, offering unparalleled opportunities for customer interaction. Join Knowbl as we delve into the transformative potential of Large Language Models (LLMs) and their role in fulfilling this long-awaited promise.
This Tech Forum session aims to dissect the fundamental distinctions between RAG (Retrieval Augmented Generation) LLMs and shed light on the nuances that differentiate these technologies in conversational AI.
Moreover, we’ll be navigating the critical compliance landscape within conversational automation. While guardrails are essential, the Knowbl team will illustrate why a compliance-approved approach necessitates more than mere guidelines, showcasing how Knowbl tackles this challenge effectively.
Amidst the conversational AI buzz, this Tech Forum seeks to transcend the hype.
Attendees will:
- Learn why leading brands are embracing this technology’s solutions.
- Uncover tangible applications, success stories, and real-world scenarios where these solutions have reshaped customer experiences for enterprises.
- Understand the differences between RAG and LLMs.
- Grasp the potential of LLMs in transforming how customers interact with brands, marking a significant shift in the dynamics of customer-brand relationships.
This enlightening keynote address will dive into the transformative impact of customer-centric strategies on business success. In this engaging talk, Tracy Sedlak, VP of Customer Success at Offerpad, unravels the pivotal role of harnessing Voice of the Customer data in shaping robust business strategies, fostering a culture of customer-centric excellence, and embracing the power of stepping outside our comfort zones to glean unparalleled insights from customers.
Discover how these invaluable insights are not just indicators but catalysts for innovation and growth, driving organizations toward a future where understanding and leveraging the customer’s voice are at the heart of every strategic decision.
You can hear more about Tracy’s brand story and leadership journey at CRS Tucson.
When you love the great outdoors, it makes sense to try and do everything you can to protect it. That’s why Arc’teryx, the high performance outdoor equipment brand, is doing its part to consider its environmental impact every step of the way. The brand is committed to incorporating sustainability and circularity into every business decision, making them integral to its core business.
Join Dave Pitsch, Vice President of Guest Services at Arc’teryx, as he addresses the brand’s unwavering commitment to delivering a premium guest experience and investing in its guests throughout the entire lifecycle of its products. The brand’s emphasis on performance, attention to detail, and dedication to environmental responsibility has garnered a strong reputation among outdoor enthusiasts.
Learn how the brand is shaping the future of guest experience, addressing crucial moments of truth, and aligning its product design, circularity initiatives, and guest services to cater to the evolving needs and expectations of its guests.
Simplifying Contact Center Processes to Drive Cost Savings and Better Experiences
The contact center industry plays a pivotal role in delivering efficient customer service and support across various sectors. However, operating a contact center can be costly, primarily due to the highly labor-intensive nature of its operations. To address this challenge, organizations are increasingly turning to automated process flows to optimize their contact center operations and achieve significant cost savings.
Join us as we share the remarkable transformation journey for PSCU, the nation’s largest credit union service organization, and how they leveraged AI and automation to simplify hundreds of workflow processes and eliminate their complicated knowledge base—all while boosting agent performance and efficiency.
By streamlining and automating data retrieval and consolidation, knowledge management, and issue escalation processes, agents can dedicate their expertise to more complex, value-added tasks. This not only amplifies their productivity but also elevates customer satisfaction by ensuring faster and more precise resolutions.
In this session, Kim West, VP Product Marketing from Uniphore, will share invaluable lessons and key takeaways to:
- Harness the power of AI to automate knowledge search and time-consuming tasks
- Ensure compliance with industry regulations through guided workflows
- Drive retention by empowering agents to focus on the customer experience
Creating a Healthy, Tech-Empowered Workplace
Technology is most effective when it’s used in the service of human beings. Join Intradiem President Jennifer Lee for a customer case study featuring Jim Simmons from Synchrony Financial. Jennifer will discuss technology-driven strategies to boost employee well-being and performance, and Jim will explain how Synchrony Bank is leveraging automation along with Thrive’s science-backed behavior change platform to provide more human-centric support to its contact center agents. Arianna Huffington, founder and CEO of Thrive Global, will share via video how Thrive embeds employee well-being into everyday workflows, which lowers stress, builds resilience and improves performance.
The Lifelong Conversation: How to Make Customer Service Your Business Using a Seamless Channel Experience
In today’s competitive business landscape, customer service has become the ultimate differentiator. It’s no longer just about resolving issues; it’s about creating meaningful connections that foster loyalty and advocacy. Channel shouldn’t matter when it comes to delighting customers. The modern customer service team needs to meet customers where they are without having their information siloed across tools or channels. This panel discussion will bring together industry experts, thought leaders and seasoned practitioners to explore effective strategies and best practices for cultivating lifelong conversations with customers. Through engaging dialogue and insightful anecdotes, the panelists will highlight the key elements contributing to making customer service the centerpiece of your business by establishing a seamless channel experience that transcends traditional boundaries.
The Era of Intelligent CX: Opportunities and Challenges
We all see it coming, an AI revolution that is more than just hype. However, figuring out how to make it work smoothly for customer service and finding the best way to learn, evaluate, and put it into action is not a walk in the park.
Join us as we examine the opportunities for leveraging AI to better understand, shape, customize, and optimize the customer journey and your overall CX. We’ll share real-world success cases and even a few cautionary tales of when things didn’t go quite as planned.
During this Shop Talk, you’ll leave understanding:
- The unique requirements and considerations for applying AI in a CX setting.
- Ways to identify opportunities for AI to bring real value to your CX organization, in hours and not years.
- The future role of an agent, and how their interaction with the customer will change in the short, medium, and long term.
Digital First, Member Always: How Navy Federal Credit Union Brings this Mantra to Life
Navy Federal Credit Union (NFCU) has always been focused on providing member-first service to more than 12 million global customers, and their frequent recognition by Forrester, KPMG, and JD Power reflects that emphasis. With the advent of more advanced technology, NFCU has worked hard to strike a balance between the high-touch interactions and digital journeys they provide to members. To guide this work, NFCU adopted a Digital First, Member Always philosophy.
In this session, we will share a glimpse into some of the ways the NFCU customer experience teams are bringing this mantra to life. Starting with the fundamentals, we will discuss key initiatives that are improving the member’s digital journey.
You will learn about:
- How NFCU got started on this journey and how the philosophy drives the organization’s focus
- Some of the new capabilities and processes NFCU has launched to achieve this balance and recent results that highlight success
- Future plans for additional digital self-service capabilities, including expansion of multi-channel bots and large language models (e.g., ChatGPT)
We hope you will join us to hear about NFCU’s exciting journey as they deliver compassionate service that strives to always put the member first!
Leading Across Generations: Understanding and Embracing Differences in the Modern Workforce
As the workforce grows and diversifies, leading across generations has become more challenging than ever before. There are now five generations in the workforce (Silent Generation, Baby Boomers, Generation-X, Millennials, Generation-Z), each with unique values, communication styles, work preferences, motivators, and technology habits. This can lead to misunderstandings and incorrect assumptions among colleagues, making it critical to welcome different perspectives and use them to lead effectively.
In this workshop, COPC Inc. will present 2023 research that illuminates the reality of generational divides in contact centers and customer experience operations.
Attendees will:
- Learn the characteristics, preferences, and drivers of each generation and their impact in the workplace
- Discuss how to gather similar insights and data from their employees
- Uncover effective leadership methods for each generation, while understanding the importance of individual management styles for each person
- Create a leadership enhancement plan based on shared research and best practices (participants can bring their own data if they have it)
- Share thoughts on how to ensure generational awareness is spread throughout the management chain down to frontline management
Join COPC and your peers to learn how to transform your leadership approach and create a culture that welcomes generational differences. Leave this session with a better understanding of how to lead and manage each generation while maintaining individual strategies for maximum success.
SmileDirectClub Facility Tour
Join Execs In The Know on Friday, September 22nd at 2 PM for an exclusive behind-the-scenes tour of SmileDirectClub’s manufacturing facility, dubbed the SmileHouse.
Your host Alvin Stokes, SmileDirectClub’s Chief Customer Contact Officer, will share how this disruptive brand streamlines the process from customer engagement to purchase decision and innovates the CX journey for their customers. He’ll delve into the fascinating connection points between customer experience and the SmileMaker Platform, which uses advanced AI technology via an app to put game-changing innovation in the palm of consumers’ hands.
Be a part of this tour to witness firsthand the impressive 3D printing technology, observe the seamless production of the brand’s clear aligners, and explore how using the power of innovation can up-level your customer experience. We can’t wait to see you there!
Unleashing the Power of Customer Data: How iRobot Leverages Customer Care to Build Lasting Relationships in a Competitive Tech Landscape
As a leading robotic vacuum cleaning company, iRobot prides itself on being a mission-driven builder that is revolutionizing the way the world cleans with consumer robots. In this thought-provoking keynote, Ledia Dilo, VP – Head of Global Customer Care and Fulfillment at iRobot, will address how the brand leverages customer-centric strategies to drive sustained growth, optimize operations, and build customer loyalty in an industry currently challenged by slowing demand, growing competition, and supply chain cost.
With a focus on optimizing operations, she’ll unveil the four strategic pillars that drive iRobot’s customer interactions, streamline onboarding experiences, and transform the contact center into an insights-driven and strategic business unit. Additionally, she’ll highlight the crucial role of the care team, their technical training, and collaborative partnerships with engineering in addressing complex product issues. Find out how iRobot leverages customer data and proactively engages with consumers to build lasting relationships. Drawing on her extensive experience, inspiring anecdotes, and metrics showcasing the success of proactive initiatives, she will demonstrate the immense value of customer-centricity in shaping the trajectory of brands to deliver win-win outcomes.
Join Ledia to gain insights on iRobot’s remarkable legacy that will inspire you to reshape your brand’s customer experience, unlock new growth opportunities, and cultivate enduring customer loyalty.
Size Is No Barrier: How Brands of Every Scale Are Navigating the AI-CX Landscape
Join our panel of experts as they delve into the world of artificial intelligence (AI) and its potential transformative impact on customer experience (CX). The revolutionary capabilities that AI offers enable brands to drive meaningful change by delivering efficiency, hyper-personalization, predictive insights, and seamless interactions to just name a few.
This thought-provoking discussion will shed light on a wide range of perspectives, various use cases, and challenges and opportunities that are specific to an organization’s size and structure when deploying AI in the current CX landscape. Our panelists will also share how they are navigating critical considerations, such as ethics, data privacy, and striking the right balance between automation and maintaining a human touch.
LOOP Insurance Replaced Their Chatbot with Generative AI and Resolution Rates Soared!
The latest AI has the potential to automate far more customer inquiries than prior generations of conversational AI. While most people are familiar with ChatGPT, few have deployed Large Language Models in customer-facing applications.
LOOP Insurance is excited to share their journey from a simple chatbot to a state of the art LLM-powered AI assistant that has yielded an impressive increase in effectiveness and CSAT.
In this session you will learn:
- How LOOP’s new AI Assistant is remarkably better than its prior generation chatbot
- What resolution rates and outcomes LOOP achieved
- How LOOP was able to harness the power of LLMs to serve customers safely
From Ordinary to Extraordinary Customer Support: A Real-World AI Success Story
Witness the transformative journey of iPostal1, the worldwide leader in digital mailbox technology, as we explore the extraordinary impact of artificial intelligence (AI) on customer service. Due to rapid growth and an influx of customers, the brand was challenged to rethink how it could maintain its high standards for an exceptional level of service.
Armed with innovative solutions and a dynamic suite of tools, iPostal1, was able to scale its operations and take control of how it managed relationships with customers without diminishing the quality of its customer service.
Join us to hear from Dan Medina, Director of Customer Service Operations at iPostal1, and Colin Crowley, CX Advisor at Freshworks, to learn how AI can empower your customers, agents, and leaders alike by:
- Deflecting queries and automating repetitive tasks
- Building efficiencies to enable improved customer resolution times and retention
- Saving agents time and getting to contacts in under a minute
- Equipping teams with valuable insights to improve operational efficiency and focus on what matters most
Achieving Cultural Alignment: Neiman Marcus Sets the Stage for Optimal Strategic Partnerships
The cultural alignment between your organization and your BPO partner has become an essential ingredient to drive performance, speed to proficiency, and a consistent customer experience across locations. When cultural alignment is measured, monitored, and continuously calibrated, both companies arrive at a greater understanding of each other’s beliefs, communication processes, and abilities—functioning with truly dynamic collaboration.
In this session, learn how Qualfon and Neiman Marcus’ cultural alignment has resulted in the ultimate strategic partnership.
- Measure your company culture using internal NPS and surveys
- Integrate your company culture into your BPOs training curriculum to produce brand ambassadors
- Discover the results that are revealed with a culturally aligned partnership
The Future of Work: Navigating the Change Curve
In a rapidly changing world, the landscape of work and business is undergoing unprecedented transformations. During his keynote, Peter Mallot, Worldwide Support Leader for Modern Life and Business Programs at Microsoft, will provide an informed perspective and timely insights at the intersection of Workforce Strategy, Culture, and Technology.
The future of work is progression, not an overnight solution. It demands a shift in corporate culture, management philosophy, and workforce adaptation, where the focus moves from reactive to proactive, from support to achievement. The path forward lies in a delicate balance between leveraging technology’s capabilities and empowering people toward greater productivity, collaboration, flexibility, and automation to enable customers to achieve more.
Join Peter to gain a deeper understanding of the engine required to drive change for an organization’s most valuable assets — its teams and its customers.
Behind the Screens: How MoviePass Is Redefining Customer Experience and Building a Moviegoer Community
In this engaging keynote address, Stacy Spikes, CEO of the nationwide movie subscription service MoviePass, will take you on a journey where customer-centricity and innovation converge to create immersive and personalized cinematic experiences for all.
Discover how MoviePass is reinventing itself post-bankruptcy, overcoming past challenges, embracing product and customer service improvements, and setting its sights on a new horizon. From navigating customer churn to building customer trust and loyalty, Stacy will share real-life examples of the pivotal moments and invaluable insights that are shaping the brand’s trajectory.
Learn why diversity and building community are not just cultural values, but smart business moves that lead to better products and greater market reach. Additionally, Stacy will delve into the potential of blockchain technology as a powerful tool to bridge the gap between moviegoers and filmmakers.
Join us for this thought-provoking and inspiring look into the future of cinema and Stacy’s ambitious plans for reinventing moviegoing for customers.
Finding the ROI of Your CX Vision
As CX leaders, we recognize that enabling a customer-centric vision holds immense potential for driving value for our organizations.
Join our esteemed panel of industry experts as we explore how CX is used to drive revenue opportunities and strong business value. The panel will unlock the key KPIs and performance metrics that leaders must be measuring and monitoring to validate and amplify the value of their CX vision. With an ever-changing technology landscape alongside evolving customer expectations, we will explore the future impacts of CX ROI and discuss the influence it will have on what we measure.
From this session you will take away:
- The CX outcomes that can drive value to your organization.
- Effective KPIs and performance metrics that demonstrate the value of your CX initiatives.
- Considerations on how to measure and evaluate your future state of CX.
What Is a Digital Worker?: Harnessing ChatGPT and Automation to Create New Strategies in CX
Enter digital workers, where AI and automation meet to support employee and customer experiences. They are changing our understanding of the future of work.
To support human employees, digital worker technology will continue to advance capabilities. The unique capabilities of Generative AI and automation are enabling CX leaders to gain a deep understanding of customer preferences, behaviors, and pain points.
Join us as we explore a real-world example of how a leading brand harnessed digital workers to revolutionize its contact center operations.
During this Shop Talk, you’ll discover:
- How digital workers can connect with customers by performing tasks guided by the power of Generative AI technology to provide consistent, on-brand CX.
- Ways to layer this technology onto your existing systems to overcome traditional tech blockers and modernize your customer care strategies.
- The tangible outcomes achieved by real-world clients, including accelerated productivity, exceptional first-contact resolution, and elevated customer satisfaction.
- How to leverage this technology in new ways to enhance agents and scale up customer service
Data-Driven Customer Insights: Leveraging Analytics to Anticipate and Exceed Customer Expectations
As artificial intelligence (AI) and machine learning (ML) technology evolve, contact centers are using them to transform customer experiences through agent assist, self-service, and conversational analytics capabilities.
Join Amazon Web Services (AWS) and Truist to discover best practices for how to leverage AI-powered technology to deliver exceptional customer experiences. In this session, you will learn about:
- Executing a connected channel strategy that delivers consistent customer experiences
- Leveraging AI/ML for real world outcomes like better self-service, agent assistance, and conversational analytics
- Turning data into insights that drive continuous improvement of your customer experience
The Agent, the Data, and Technology: Three Pillars for Enabling CX Personalization
With the global CX personalization market forecast to hit $11.6 billion by 2026, it is imperative that brands embed true personalization opportunities across the service journey. Facilitation begins with bi-directional omnichannel engagement that will require cutting-edge technology, insightful analytics, and advanced agent enablement.
This incredible panel will showcase agents alongside their business leaders in what is sure to be a powerful conversation on the approach needed to radically transform customer relationships. Join us as we unpack and explore critical insights from two unique perspectives on:
- What agents need to enable next-level personalization
- The influential role of agent empowerment in reaching CX goals
- How advanced analytics will unlock new opportunities
- Reaching the goal of moving Customer Service from a cost center to a profit center
- The role of technology and how it sets frontline agents up to create even more meaningful relationships with customers
Ignite the Leader Within: Embracing the Power of Inspiration for Extraordinary Results
Inspiration is a catalyst for greatness. It transcends the mundane and ordinary, breaking through conventional boundaries to unleash the full potential of individuals and teams. In this captivating keynote, Carolyne M. Truelove, Vice President, Reservations and Customer Relations at American Airlines, will unveil the immense power of inspiration and its ability to ignite a drive for exceptional results and lead transformative change.
Discover the extraordinary possibilities that lie within you as a leader. Everything a leader does requires connection, including vision, strategy, and execution. Learn the art of connected leadership, fostering a culture of open-mindedness, and leveraging your leadership to navigate through the ever-evolving business landscape. When inspiration permeates every aspect of an organization, magic happens. Join Carolyne for an unforgettable keynote and unlock the secret to inspiring others into action.
Building Trust in Generative AI
Generative AI brings forth a new realm of possibilities for enhancing the customer experience. However, its propensity to occasionally deliver inaccurate or nonsensical information — a phenomenon known as a hallucination — could potentially impact hard-won customer loyalty. To ensure its success, generative AI has to solve problems and respond in a way that is accurate, helpful and free from toxicity and bias.
Join AI experts from TELUS International, a leading digital customer experience provider, for a Tech Forum exploring:
- The emerging technologies and processes that are being deployed to increase the accuracy of generative AI models.
- Potential legislation, similar to the AI Bill of Rights, being proposed in the wake of the generative AI explosion.
- Consumer perception of AI-generated content, including accuracy, completeness, attribution and age-appropriateness.
- And more!
How to Cultivate a Culture of Well-Being for a Resilient Workforce
Companies today require a robust well-being strategy to have a material impact on the growing crisis of workplace mental health and business resilience. Join us for this interactive session as we explore the Four Building Blocks of Emotional Intelligence. We’ll be exploring how to intentionally cultivate learning, development, and leadership strategies that focus on mindfulness and emotional intelligence, and how they are enhanced by dynamic neuroinsights and AI technology.
Key takeaways:
- Learn how well-being helps to drive retention, effectiveness, and efficiency gains throughout the workforce.
- How well-being technology programs leverage AI to assist with the timely delivery of well-being interventions.
- Ways to deeply integrate holistic system design and well-being practices across the employee lifespan.
The Conversational AI Divide
There is a great divide emerging in customer experience (CX) and leaders are being presented with the opportunity to blaze a trail forward with Conversational AI to set themselves apart in their service experience.
Conversational AI presents the opportunity for companies to harness new technology in a low-risk, high-impact environment. Embracing these new possibilities makes it possible for CX leaders to deliver enhanced and effective service at scale.
During this Tech Forum, you will be guided through the steps and considerations needed to build your very own AI assistant. Participants will walk away appreciating how simple and risk-free it can be to deliver big CX wins.
Join us as we explore:
- The universal presence of AI assistants: Understanding how AI assistants are becoming ubiquitous, shaping the future of customer interactions across industries.
- Building better customer experiences with reduced levels of effort: Discovering strategies to leverage Conversational AI to streamline and enhance the customer journey, delivering seamless experiences that require minimal effort from customers.
- The ease of creating your very own virtual AI assistant: Exploring the possibilities of building custom AI assistants without the need for extensive coding or AI expertise, opening new avenues for innovation and personalized experiences.
- Uncovering the distinct advantages and untapped potential that can be leveraged by embracing Conversational AI and staying at the forefront of this evolving landscape.
Don’t miss this opportunity to bridge the Conversational AI divide and unlock the full potential of AI assistants in revolutionizing your CX.
Improving EX in a Remote Workforce
In today’s remote work environment, organizations must not only hire the right talent but must also leverage technology to effectively manage and improve the employee experience (EX) throughout the agent life cycle.
To boost agent experience and reduce cognitive load, leading organizations are:
- Using job simulation previews to set realistic expectations
- Helping accelerate onboarding and training through micro-module learning and personalized AI avatars
- Managing agent stress and anxiety with rapid employee feedback and real-time reporting
- Applying generative AI across EX to drive improved satisfaction
In this 90-minute session, we’ll explore how to best empower remote employees and motivate them to foster collaboration amongst themselves as well as with managers. With effective strategies in place, companies will see higher ESAT, engagement, and retention from happy and productive team members.
AI Simulation Roleplay Training for Speed to Proficiency in the New Hybrid Workforce
As you build onboarding for new hires, getting your agents to a place of confidence and proficiency can often be a challenge, especially in a hybrid workforce environment.
In this interactive session, we will reveal key findings on CX preferences designed for today’s consumers and what experiences they truly expect when contacting your brand for support. Find out why driving higher speed to proficiency with new hires is the top metric for delivering the experience your customers expect and deserve.
The hybrid workforce is here to stay. Learn insights into why companies are losing customers and market share by not having effective onboarding. While companies shifted to ‘virtual learning’ due to need, most lack a cohesive and scalable training and onboarding strategy for new hires in a global hybrid workforce.
In this session you’ll learn:
- The most effective methods to onboard new hires in a global hybrid workforce
- Best practices from your peers that are leading the charge in driving speed to proficiency
- Key findings on CX preferences impacting your business
- How to best scale onboarding consistency and accountability
- Which innovative technologies can be leveraged to achieve key outcomes
JACK MEEK
Vice President, US Care Support Operations, GoDaddy
Jack Meek currently leads customer care operations at GoDaddy, the world’s largest services platform for entrepreneurs around the globe. GoDaddy’s mission is to empower their worldwide community of 20+ million customers — and entrepreneurs everywhere — by giving them all the help and tools they need to grow online. With 21M+ customers worldwide and 84M+ domain names under management, GoDaddy is the place folks come to name their idea, create a compelling brand and a great looking website, attract customers with digital and social marketing, and manage their work. Jack is responsible for developing and executing the future global care strategy that will continue to differentiate GoDaddy as the advocate of small business success throughout the world.
Jack is an influential change leader who has a passion for technology, innovation, and seeing people succeed. His foundational expertise and passion for building a solid employee and customer centric culture started at MCI where he was repeatedly recognized for record-breaking performance & leadership achievements. He then went on to help build and consolidate large-scale customer service and sales organizations at in the Telecom and Retail Energy space. Jack has successfully transformed and maintained customer operations and sales organizations of all sizes and across many different industries. He spent several years as a senior leader in the Business Process Outsourcing (BPO) industry helping multiple clients such as Verizon, Sony, and T-Mobile achieve their customer outcome goals.
JEFF MYERS
Vice President and General Manager Customer Care, SiriusXM
Jeff is a passionate advocate for Customers and designing frictionless experiences for them. He began his career in Marketing in 1991, as a call center agent, while attending the University of Wisconsin, Madison and studying Communication Theory and Research. The first eight years of his career included multiple call center operational roles. Following his first eight years in operations, Jeff spent 10 years in the Omnicom family in both the US and the UK/Europe with multi-channel responsibility for Client Services and Program Strategy in a variety of verticals including Telecom, Energy, Media and Non-Profit. He currently serves as the VP and GM of Listener Care for SiriusXM with responsibility for all live customer interactions. His scope includes partnerships with 16 BPOs, in nine geographies, representing more than 60 support center locations.
Bill Colton
Co-Founder and CEO, Global Telesourcing
Bill Colton is the Co-Founder and CEO of Global Telesourcing, a premium provider of digital and voice customer experience and sales solutions for some of the largest and most recognizable brands in the US. Using native-speaking English agents who spent their formative years living in the US, their workforce is as bi-cultural as they are bi-lingual. Global Telesourcing serves clients from centers in both Monterrey and Leon, Mexico, as well as work-from-home.